Special Features
Special features that no other Neural Network software has:
27 activated functions
Users can choose different activated functions in different layers
Easy to use, friendly user interface
Available library in C++ and C#
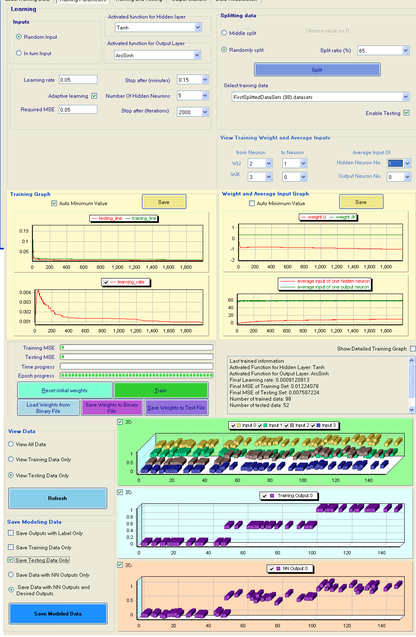
Extra Resources
User guide:
- In English
- In Vietnamese
Self-Organizing Map and MLP Neural Network – A Practical Use:
- In English
- In Vietnamese
Sample Codes - Example of using Spice-MLP Neural Networks
// MLP_Data_Modeling.cpp
// How to compiler
// g++ MLP_Data_Modeling.cpp mlpsom_source/spice_mlp_lib.cpp -lm -O3 -ffast-math -o s.x
// source /opt/intel/system_studio_2018/bin/compilervars.sh intel64
// icpc MLP_Data_Modeling.cpp mlpsom_source/spice_mlp_lib.cpp -lm -O3 -ffast-math -o s.x
// $ ./s.x
#include <omp.h>
#include <iostream>
#include <stdio.h>
#include <stdlib.h>
#include <math.h>
#include <iostream>
#include <fstream>
#include <string>
#include <time.h>
#include <fstream>
#include <cstdio>
#include "mlpsom_include/spice_mlp_lib.h"
using namespace std;
using namespace SpiceNeuro;
struct MlpTrainingSet
{
double **fInputs; //input data
double **fOutputs; //output data
char (*strLabel)[255]; //label of the dataset
int nDataSet; //number of datasets
int nInputs; //number of Inputs
int nOutputs; //number of Outputs
};
void InitMlpDataSet(MlpTrainingSet *DataSets, int nTrainingDataSet, int nInputs, int nOutputs);
void FreeDataSet(MlpTrainingSet *DataSets);
int findMaxIndex(double *data, const int nData);
// find max in output of a neuron
int findMaxIndex(double *data, const int nData)
{
float max = data[0];
int ind = 0;
for (int i = 1; i < nData; i++)
{
if (max < data[i]){
max = data[i];
ind = i;
}
}
return ind;
}
//initialize new dataset with number of datasets = nTrainingDataSet
void InitMlpDataSet(MlpTrainingSet *DataSets, int nTrainingDataSet, int nInputs, int nOutputs)
{
DataSets->fInputs = new double*[nTrainingDataSet];
DataSets->fOutputs = new double*[nTrainingDataSet];
DataSets->strLabel = new char[nTrainingDataSet][255];
for (int i = 0; i < nTrainingDataSet; i++)
{
DataSets->fInputs[i] = new double[nInputs];
DataSets->fOutputs[i] = new double[nOutputs];
}
DataSets->nDataSet = nTrainingDataSet;
DataSets->nInputs = nInputs;
DataSets->nOutputs = nOutputs;
return;
}
void FreeDataSet(MlpTrainingSet *DataSets)
{
for (int i = 0; i < DataSets->nDataSet; i++)
{
delete [] DataSets->fInputs[i];
delete [] DataSets->fOutputs[i];
}
delete [] DataSets->fInputs;
delete [] DataSets->fOutputs;
delete [] DataSets->strLabel;
return;
}
int CreateTrainingDataFromCsv(char *txtFilename, int nData, int nInputs, int nOutputs)
{
printf("creating %s\n", txtFilename);
MlpTrainingSet newData;
InitMlpDataSet(&newData, nData, nInputs, nOutputs); // initialize
FILE *f = fopen(txtFilename, "r");
char tmp[255];
/*
// data without header? comment it
for (int i = 0; i < nInputs + nOutputs + 2; i++){
fscanf(f, "%s", tmp);
}
*/
for (int j = 0; j < nData; j++)
{
// data without ID, comment ID
// fscanf(f, "%s", tmp); // ID
for (int i = 0; i < nInputs; i++){
fscanf(f, "%s", tmp);
newData.fInputs[j][i] = atof(tmp);
}
for (int i = 0; i < nOutputs; i++){
fscanf(f, "%s", tmp);
newData.fOutputs[j][i] = atof(tmp);
}
fscanf(f, "%s", tmp); // label
sprintf(newData.strLabel[j], tmp); // assign label for the i-th dataset, please remember length of the label is 255
}
fclose(f);
char fname[255];
sprintf(fname, "%s_read.txt", txtFilename);
f = fopen(fname, "w");
for (int j = 0; j < nData; j++){
for (int i = 0; i < nInputs; i++){
fprintf(f, "%f ", newData.fInputs[j][i]);
}
for (int i = 0; i < nOutputs; i++){
fprintf(f, "%f ", newData.fOutputs[j][i]);
}
fprintf(f, "%s \n", newData.strLabel[j]);
}
fclose(f);
sprintf(fname, "%s.dat", txtFilename);
// write data to binary file
FILE *fout = fopen(fname, "wb");
fwrite(&newData.nDataSet, sizeof(int), 1, fout);
fwrite(&newData.nInputs, sizeof(int), 1, fout);
fwrite(&newData.nOutputs, sizeof(int), 1, fout);
for (int j = 0; j < newData.nDataSet; j++)
{
fwrite(newData.fInputs[j], sizeof(double), newData.nInputs, fout);
fwrite(newData.fOutputs[j], sizeof(double), newData.nOutputs, fout);
fwrite(newData.strLabel[j], sizeof(char), 255, fout);
};
fclose(fout);
FreeDataSet(&newData);
return 0;
}
// create data from csv file
int main_CreateTrainingDataFromCsv()
{
CreateTrainingDataFromCsv("TrainingData_V3-sized/Boxes.txt", 474, 36*54, 33);
return 0;
}
char label[33][24] =
{
"0-clear",
"0-unclear",
"1-clear",
"1-unclear",
"2-clear",
"2-unclear",
"7-clear",
"7-unclear",
"A-clear",
"A-unclear",
"B-clear",
"BI-clear",
"BI-unclear",
"C-clear",
"C-unclear",
"D-clear",
"D-unclear",
"E-clear",
"F-clear",
"F-unclear",
"G-clear",
"G-unclear",
"H-clear",
"J-clear",
"K-clear",
"L-clear",
"naka-clear",
"naka-unclear",
"RU-clear",
"RU-unclear",
"T-clear",
"T-unclear",
"Y-clear" };
int main()//_train_MLP()
{
int nHiddens = 33;
char dataFile[255], modelingFile[255], fClassInClass[255], fClassInClassBrief[255];
char *folder = "data/";
sprintf(fClassInClass, "%s/ClassInClass.txt", folder);
sprintf(fClassInClassBrief, "%s/ClassInClassBrief.txt", folder);
sprintf(dataFile, "%s/Boxes.txt.dat", folder);
printf("datafile = %s\n", dataFile);
SpiceMLP *MLP = new SpiceMLP("Example of MLP", 36 * 54, nHiddens,33); // initialize a MLP with 36*54 input, 33 hiddens and 33 outputs
printf("MLP->DataSets.nInputs = %i\n", MLP->DataSets.nInputs);
printf("MLP->DataSets.nOutputs = %i\n", MLP->DataSets.nOutputs);
printf("MLP->GetLayers.GetInputNeurons = %i\n", MLP->GetLayers.GetInputNeurons());
printf("MLP->GetLayers.GetHiddenNeurons = %i\n", MLP->GetLayers.GetHiddenNeurons());
printf("MLP->GetLayers.GetOutputNeurons = %i\n", MLP->GetLayers.GetOutputNeurons());
MLP->InitDataSet(474, 36 * 54, 33); // initialize data structure
MLP->DataSplitOptions.iMiddle = 20;
// set training parameters
MLP->TrainingParam.bAdaptiveLearning = false;
MLP->TrainingParam.bRandomInput = true;
MLP->TrainingParam.bEnableTesting = true;
MLP->TrainingParam.fLearningRate = 0.0005f;
MLP->TrainingParam.fMseRequired = 0.0;
// AF_Gaussian->AF_Sigmoid is ??
MLP->TrainingParam.HiddenActivatedFunction = SpiceNeuro::AF_HyperTanh;//AF_HyperTanh, AF_Sigmoid
MLP->TrainingParam.OutputActivatedFunction = SpiceNeuro::AF_Sigmoid;
printf("MLP->LoadTrainingData(dataFile) = %s\n", dataFile);
MLP->LoadTrainingData(dataFile);
printf("MLP->LoadTrainingData(dataFile) = %s OK\n", dataFile);
//MLP->NormalizeInputData(Normalization_Linear, 0, 1);
//asign split option, then do split data
//MLP->DataSplitOptions.iRandomPercent = 70;
//MLP->DataSplitOptions.SplitOptions = SpiceNeuro::Split_Random;
//MLP->DataSplitOptions.SplitOptions = SpiceNeuro::Split_Middle;
//MLP->SplitData();
MLP->AssignTrainingData(SpiceNeuro::Use_AllDataSets); // Use_FirstSplittedDataSets, ot Use_AllDataSets
//Train the MLP in some iterations
double fTimeStart, fTimeEnd, fTimeLast=0;
fTimeStart = MLP->fGetTimeNow();
// train mlp in 1000 iterations
printf("start to train\n");
int nIteration = 1000;
MLP->ResetWeights();
double fFinal = MLP->DoTraining(nIteration);
fTimeEnd = MLP->fGetTimeNow();
printf("1000 iteration take %f (%f)sec, fFinal = '%f'\n", fTimeEnd - fTimeLast, fTimeEnd - fTimeStart, fFinal);
fTimeLast = fTimeEnd;
//Now, test the modeling data
double *dOneSampleInputs = new double[MLP->GetLayers.GetInputNeurons()];
double *dOneSampleReponse = new double[MLP->GetLayers.GetOutputNeurons()];
double **newResponse = new double*[MLP->DataSets.nDataSet];
for (int i = 0; i < MLP->DataSets.nDataSet; i++){
newResponse[i] = new double[MLP->GetLayers.GetOutputNeurons()];
}
for (int j = 0; j < MLP->DataSets.nDataSet; j++)
{
//Get inputs of one dataset
for (int i = 0; i < MLP->GetLayers.GetInputNeurons(); i++){
dOneSampleInputs[i] = MLP->DataSets.fInputs[j][i];
}
MLP->ModelingOneDataSet(dOneSampleInputs, dOneSampleReponse);
//Get modeling value of the one dataset
for (int i = 0; i < MLP->GetLayers.GetOutputNeurons(); i++){
newResponse[j][i] = dOneSampleReponse[i];
}
}
//print modeling data to screen and file
sprintf(modelingFile, "%s/Boxes.modeling_%i-iter-%i-hiddens.csv", folder, nIteration, nHiddens);
FILE *fc = fopen(modelingFile, "w");
fprintf(fc, "strLabel[j], label[indDesired], indDesired, indModeling, label[indModeling], newResponse[][indModeling]\n");
for (int j = 0; j < MLP->DataSets.nDataSet; j++)
{
int indDesired = findMaxIndex(MLP->DataSets.fOutputs[j], MLP->DataSets.nOutputs);
int indModeling = findMaxIndex(newResponse[j], MLP->DataSets.nOutputs);
fprintf(fc, "%s,%s,%i,%i,%s,%f,", MLP->DataSets.strLabel[j], label[indDesired], indDesired, indModeling, label[indModeling], newResponse[j][indModeling]);
for (int i = 0; i < MLP->GetLayers.GetOutputNeurons(); i++) {
fprintf(fc, "%f, ", newResponse[j][i]);
}
fprintf(fc, "\n");
}
fclose(fc);
// save training & testing error
sprintf(modelingFile, "%s/Boxes-%i-iter-%i-hiddens-error.csv", folder, nIteration, nHiddens);
fc = fopen(modelingFile, "w");
for (int i = 0; i < nIteration; i++)
{
fprintf(fc, "%i,%f,%f\n", i, MLP->GetErrors.GetTrainingMSE(i), MLP->GetErrors.GetTestingMSE(i));
}
fclose(fc);
//*********************************
/*
// find the matched output
// search for the output
const int nOutputs = 10;
// match of output[op] on all classes
int nMatched_I_in_J[nOutputs][nOutputs];
int nSampleOfClass[nOutputs];
for (int j = 0; j < nOutputs; j++) {
nSampleOfClass[j] = 0;
for (int i = 0; i < nOutputs; i++) {
nMatched_I_in_J[j][i] = 0;
}
}
// calculate nMatched class in class
for (int op = 0; op < MLP->DataSets.nOutputs; op++)
{
for (int j = 0; j < MLP->DataSets.nDataSet; j++)
{
int indDesired = findMaxIndex(MLP->DataSets.fOutputs[j], MLP->DataSets.nOutputs);
if (op != indDesired)
continue;
int indModeling = findMaxIndex(newResponse[j], MLP->DataSets.nOutputs);
nSampleOfClass[op] += 1;
nMatched_I_in_J[indDesired][indModeling] += 1;
}
}
// save class in class to file
fc = fopen(fClassInClass, "w");
fprintf(fc, "Class In Class\n");
for (int class1 = 0; class1 < MLP->DataSets.nOutputs; class1++)
{
for (int class2 = 0; class2 < MLP->DataSets.nOutputs; class2++)
{
if (class1 == class2)
fprintf(fc, "Class %i on Class %i (correct)\n", class1, class2);
else
fprintf(fc, "Class %i on Class %i (mistake)\n", class1, class2);
for (int j = 0; j < MLP->DataSets.nDataSet; j++)
{
int indDesired = findMaxIndex(MLP->DataSets.fOutputs[j], MLP->DataSets.nOutputs);
if (indDesired != class1)
continue;
// class1 on class2
int indModeling = findMaxIndex(newResponse[j], MLP->DataSets.nOutputs);
if (indModeling != class2)
continue;
fprintf(fc, "%s\n", MLP->DataSets.strLabel[j]);
}
}
}
fclose(fc);
FILE *f = fopen(fClassInClassBrief, "w");
printf("modeling for %s\n", dataFile);
fprintf(f, "Class,Total,Correct,");
for (int j = 0; j < nOutputs; j++)
fprintf(f, "on_Class_%i,", j);
fprintf(f, "\n");
for (int j = 0; j < nOutputs; j++) {
fprintf(f, "Class_%i,%i,", j, nSampleOfClass[j]);
fprintf(f, "%i,", nMatched_I_in_J[j][j]);
for (int i = 0; i < nOutputs; i++) {
fprintf(f, "%i,", nMatched_I_in_J[j][i]);
printf("%i,", nMatched_I_in_J[j][i]);
}
fprintf(f, "\n");
printf("\n");
}
fclose(f);
*/
//*********************************
delete [] dOneSampleInputs;
delete [] dOneSampleReponse;
for (int i = 0; i < MLP->DataSets.nDataSet; i++)
delete [] newResponse[i];
delete [] newResponse;
delete MLP;
printf("Press Enter to exit\n");
getchar();
return 0;
}
Sample Codes - Activated functions in Spice-MLP Neural Network
AF_Linear, // 1. y = a*x AF_Identity, // 2. y = x AF_Sigmoid, // 3. y = (1.0 / (1.0 + exp(-x))) AF_HyperTanh, // 4. y = (3.432 / (1 + exp(-0.667 * x)) – 1.716) AF_Tanh, // 5. y = (exp(x) – exp(-x)) / (exp(x) + exp(-x)) AF_ArcTan, // 6. y = atan(x) AF_ArcCotan, // 7. y = pi/2 – atan(x) AF_ArcSinh, // 8. y = log(x + sqr(x * x + 1)) AF_InvertAbs, // 9. y = x/(1+abs(x)) AF_Scah, // 10. y = scah Hyperbolic Secant, ex. scah(x) AF_Sin, // 11. y = sin(x) AF_Cos, // 12. y = cos(x) AF_ExpMinusX, // 13. y = exp (-x) AF_ExpX, // 14. y = exp (x) | AF_Cubic, // 15. y = x*x*x AF_Quadratic, // 16. y = x*x AF_SinXoverX, // 17. y = sin(x)/x AF_AtanXoverX, // 18. y = atan(x)/x AF_XoverEpx, // 19. y = x/exp(x) == x *exp(-1.0 * x) AF_Gaussian, // 20. y = exp(-x*x) AF_SinGaussian, // 21. y = 3*sin(x)*exp(-x*x) AF_CosGaussian, // 22. y = cos(x)*exp(-x*x) AF_LinearGaussian, // 23. y = 2.5*x*exp(-x*x) AF_QuadraticGaussian, // 24. y = x*x*exp(-x*x) AF_CubicGaussian, // 25. y = 3*x*x*x*exp(-x*x) AF_XsinX, // 26. y = x*sin(x) AF_XcosX, // 27. y = x*cos(x) |